An Introduction to Statistical Learning - in Python
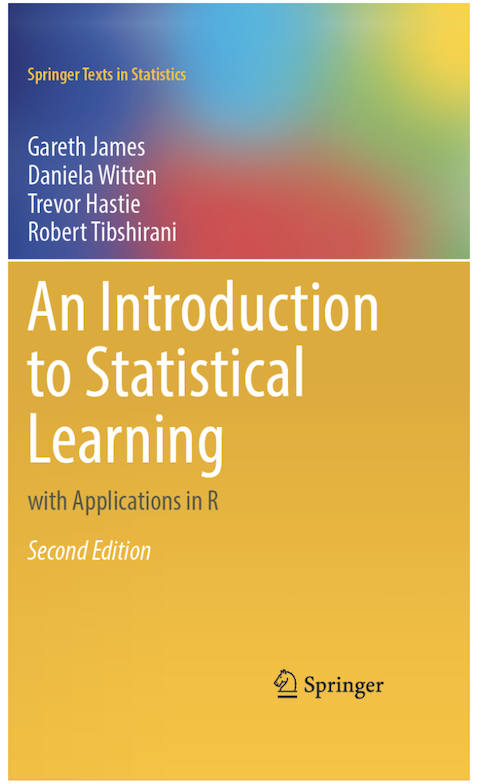
The day we have all been waiting for is here! The authors of An Introduction to Statistical Learning with Applications in R have finally launched the Python version of the book. I am a big fan of this material, as well as the FREE online course made available by the authors (you can find it in here ). Honestly, there is no better introduction to Machine Learning with such a solid footing in statistics as this one. The book contained exercises and examples in R, and now they just released a Python version of it!!! Chapter 10, on Deep Learning, was actually slightly changed to use PyTorch instead of Tensorflow (as it was done in the previous R version). When I was studying with this book, I implemented a Tensorflow Python version of the labs and exercises they made available in R. If you are curious and wants to check the Tensorflow Python version of the Deep Learning chapter you can find it in my github .